Data Privacy & Data Governance Solutions for Enterprise Healthcare Organizations
We help reduce operating costs, improve patient outcomes, create unified patient views, and speed time to discovery. How can we help you?
Health
Systems
We help health authorities organize & protect patient data across silos.
Clinics &
Research
Aggregate distributed data & make it accessible to research teams.
Drug &
Pharma
We shorten the time to find powerful insights that lead to new treatments.
Insurance
& Payers
Reduce costs by enabling data-driven decisions & finding inefficiencies.
Healthcare Data Solutions
PHEMI’s solutions are ideal for health authorities, health research centers, hospitals, and pharmaceutical companies. We can help virtually any organization in the Healthcare industry.
Privacy and Data Governance are built into the core of our solutions and are never an add-on. This makes protecting sensitive patient data a breeze.
From emails and images to CT scans and multiomics/genome data, our solution can handle large sets of structured and unstructured data with ease.
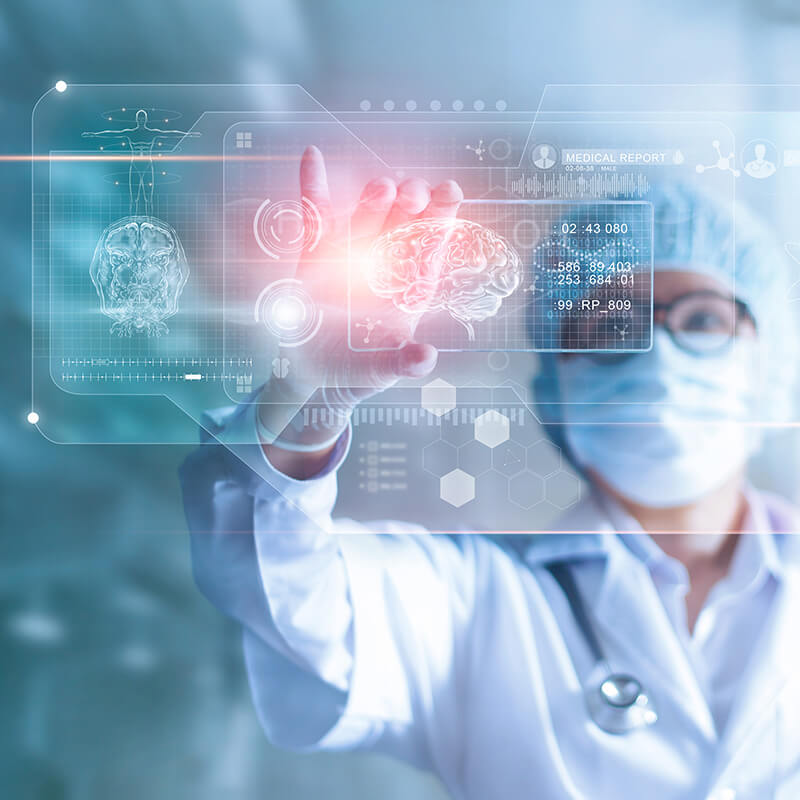
Benefits for Healthcare Organizations
Enable Precision
Medicine
Our solution maximes access to all of the allowed data to be made available for analytics and AI/ML applications.
Find Systemic
Inefficiencies
Healthcare systems struggle with hidden inefficiencies which some independent studies estimate to range from 18%-35%. The PHEMI platform enables healthcare organizations to collect & organize their data so systemic inefficiencies are easy to discover.
Improve Overall
Service Quality
By enabling access to distributed data across departmental silos without compromising privacy, PHEMI helps healthcare organizations become data-driven, improve service quality, and increase competitiveness.
Use Cases for Healthcare
AS SEEN IN
Sign Up For Everything
Data-Related:Tips, White Papers, Opinion Pieces, Webinar Invitations & News
Email use governed by our Privacy Policy
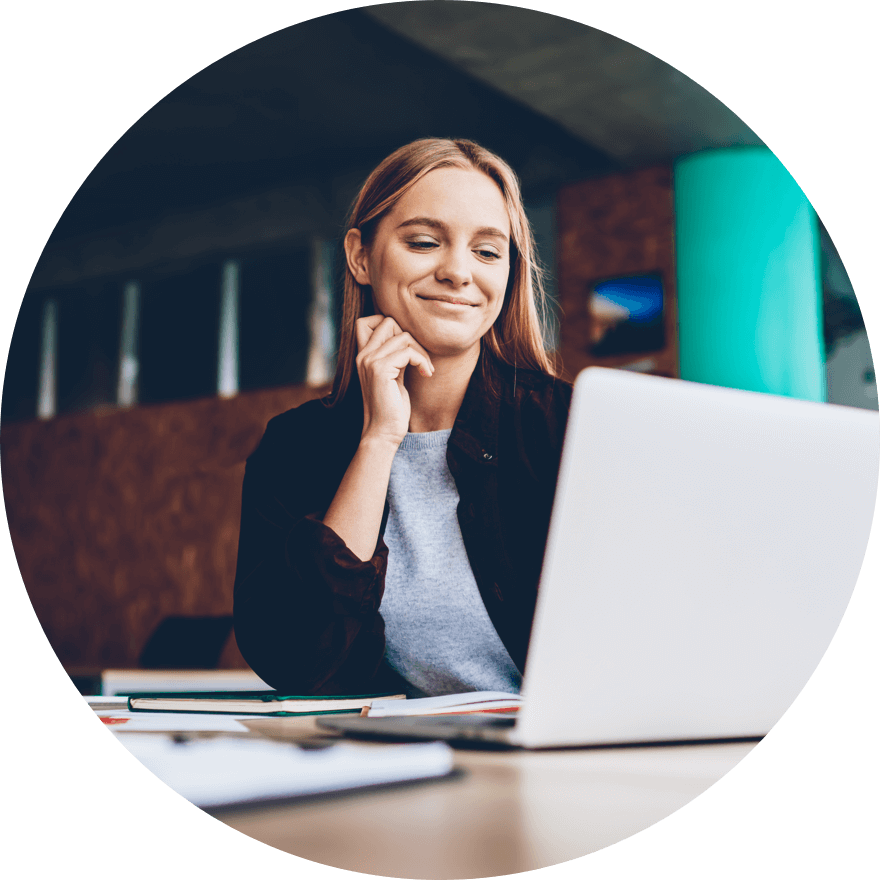